Abstract
The study leverages the Human Action Recognition (HAR) dataset, which can be accessed here. Alongside this documentation, the trained models and associated Jupyter Notebook (.ipynb) files have been attached for reproducibility and further exploration.
Deep Learning for Human Action Recognition
Objective
The goal of this project is to develop a Convolutional Neural Network (CNN) model to classify human activities from images. The model is trained to recognize and label activities from 15 predefined categories based on visual content.
Dataset
The dataset comprises over 12,000 labeled images categorized into 15 human activity classes:
- Calling
- Clapping
- Cycling
- Dancing
- Drinking
- Eating
- Fighting
- Hugging
- Laughing
- Listening to Music
- Running
- Sitting
- Sleeping
- Texting
- Using Laptop
Each class contains 840 training images, and the dataset is balanced with no missing or duplicate values.
Here are some sample images from the dataset:
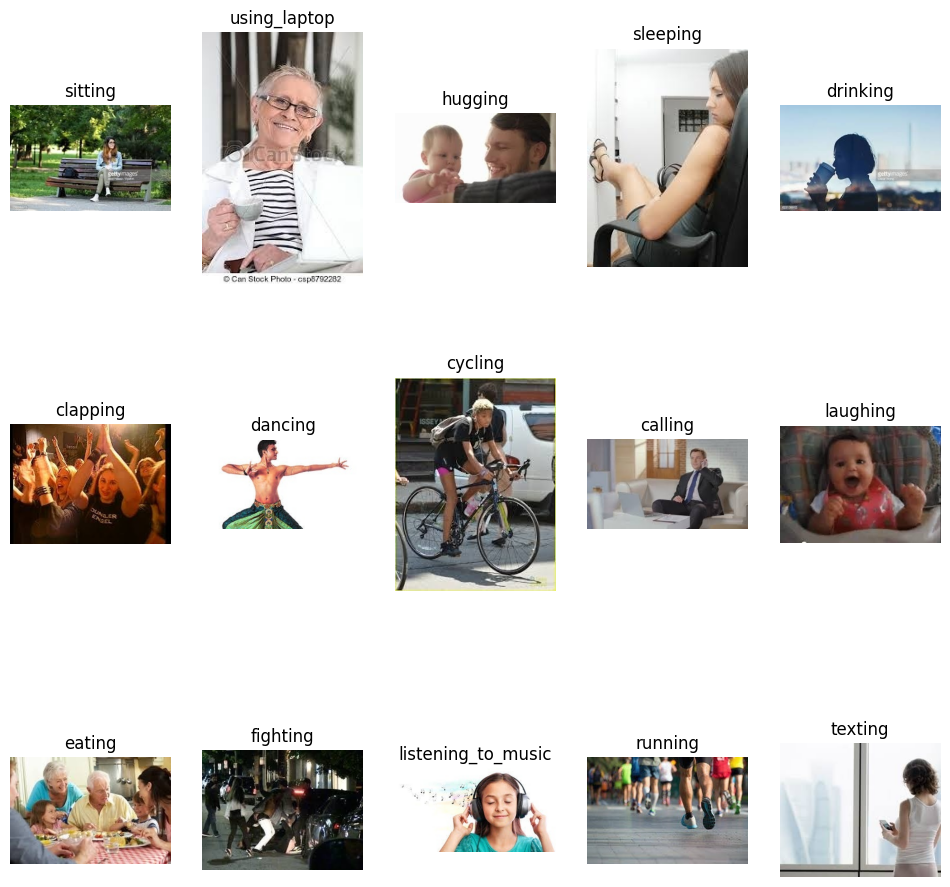
Methodology
1. Data Exploration
- Inspect dataset structure (e.g., number of classes, number of images per class).
- Visualize sample images from each class.
- Check image dimensions and label distributions.
2. Data Preprocessing
- Resize images to 128x128 pixels.
- Normalize pixel values to [0,1].
- Encode labels and split data into training (80%) and validation (20%) sets.
- Apply data augmentation (e.g., rotations, zoom, flips).
- Preprocess test data similarly.
3. CNN Model Design
- Input Layer: (128, 128, 3).
- Convolutional Blocks:
- 32, 64, 128, 256 filters with (3x3) kernels, ReLU activation, and max-pooling.
- Dense Layers:
- 512 and 256 neurons with ReLU and dropout (rate: 0.5).
- Output Layer: Softmax activation for 15 classes.
- Compilation: Adam optimizer (learning rate: 0.0001), sparse categorical cross-entropy loss, and accuracy metric.
4. VGG16-Based Model Design
- Feature Extractor: Pre-trained VGG16 (ImageNet weights, frozen base).
- Dense Layers:
- Fully connected layers with 512 neurons and ReLU activation.
- Output Layer: Softmax for classification.
- Fine-tuned the model on the dataset.
5. Model Training and Evaluation
- Trained CNN and VGG16 models on the processed dataset.
- Validation metrics were monitored for overfitting.
Results
The performance of the models is summarized in the table below:
Metric |
CNN Model |
VGG Model |
Training Accuracy |
79.44% |
81.17% |
Validation Accuracy |
54.05% |
46.91% |
Final Training Loss |
0.634 |
0.548 |
Validation Loss |
1.835 |
2.560 |
Test Accuracy (from Contest) |
56.53% |
48.29% |
Practice Rank |
8th |
N/A |
Usage
- Clone this repository.
- Load the
ipynb
files in Jupyter Notebook or Google Colab.
- Ensure you have downloaded the HAR dataset from the provided link.
- Follow the code to train or evaluate the models.
Project Structure
├── images
│ ├── sample_image.png
├── best_cnn_model.keras
├── best_vgg_model.keras
├── cnn_model_test.csv
├── vgg_model_test.csv
├── README.md
└── har-detection-testing-accuracy-56.ipynb
Acknowledgments
This project demonstrates the application of deep learning techniques in human activity recognition using image data, highlighting both challenges and potential improvements for practical deployment.